Metadata
Data documentation
In order to make research data more findable and comprehensible, documentation of data is essential. It considerably facilitates the further use of the data and enables reproducibility. Well-documented data will be used and cited more often, which will increase the reputation of the creator. Documentation is also important with regard to the subsequent usability and traceability of the data for your own work. Over time, details might be forgotten, so it is recommended to document the data while working on it. read more
Basic contents of a documentation include:
- description of the research project
- project goals
- hypotheses
- detailed information on data collection (methods, units, time periods, locations, technology used)
- measures for data cleansing
- structure of data and their relationships to each other
- explanation of variables, labels and codes
- differences between versions
- information on access and terms of use
What is metadata?
Metadata refers to structured data that contains information on other data – “data about data”. They are stored either independently of or in combination with the data they describe. There is a distinction between content-related and technical metadata. They form a specific subset of the documentation data and serve primarily to make the data findable, including in library reference systems. In order to make them machine-readable, for example in Semantic Web applications, they are often stored in XML format.
Metadata standards
Standardisation of metadata vocabulary is necessary to improve findability of the data and to provide interoperability. The linking of the metadata will ensure this. Furthermore, standards allow a uniform description of similar data sets in terms of content and structure.
Metadata standards contain a defined selection of information which is necessary to find and identify these data. This does not necessarily guarantee a reusability of the data (compare section on documentation). Among the most common bibliographic interdisciplinary metadata standards are Dublin Core, DataCite Metadata Schema, and MARC21.
Discipline-specific metadata standards
Since each scientific community has its own requirements, different discipline-specific metadata standards are also being developed. For example, in the social and economic sciences the Data Documentation Initiative (DDI) standard is frequently used, while in the natural sciences the ICAT scheme or the Crystallographic Information Framework are used.
An overview on discipline-specific metadata standards is available, for example, on the pages of the British Digital Curation Centre49 and in an overview of the Research Data Alliance.
(Biernacka, K., Bierwirth, M., Buchholz, P., Dolzycka, D., Helbig, K., Neumann, J., Odebrecht, C., Wiljes, C. and Wuttke, U. (2020). “Train-the-Trainer Concept on Research Data Management” Version 3.0. Berlin, DOI: https://doi.org/10.5281/zenodo.4071471, Creative Commons Attribution 4.0 International)
Duration: 14:30 mins
Content: Research Data and their Metadata” is an educational video on research data management. The video briefly explains the concept of metadata and where in the research data lifecycle they become important.
Schmitz, D., Hausen, D., Trautwein-Bruns, U. (2018). “Research data and their metadata” RWTH Aachen University, DOI: 10.18154/RWTH-2019-10060
License: CC BY 4.0
Welcome to our series on research data management! Today's topics are research data and their metadata. So, we are talking about, mainly, the metadata, so what metadata means and what metadata occur, typically, within research data context. We again refer to our fake science example on squirrel research. So within this project Luise Leader has built a theoretical model to look at the population dynamics of squirrels, and she has compared her theoretical ideas with concrete data gatherings from Frank Forscher in Germany and Rachel Research in Great Britain. When she looks back at this project, she remembers that it, of course, was successful but, on the other hand, that there have been quite some data management issues. And the main point in regard to this data management was that she didn't prescribe the other researchers how to do their data management, how to do their data gathering. And that was really something that, well, caused some problems.
Unfortunately Frank and Rachel gathered the data quite differently. Frank Forscher built on the many projects that he has done before. So, for example, he builds up an Excel file for each year where the different sheets represent the different federal states of Germany and you have a row for each month and you have columns then for the number of brown squirrels and the number of black squirrels. So, that was, in a way, his approach. Now Rachel. So, she travelled around a lot, moving from one location to the others, talking to the rangers, and from this it became obvious that it might make sense to organize her data on files that are collected for each month. And where you have the rows representing the different countries like Scotland, Wales and England, where she doesn't use the countries, but some country code that has been defined in a separate file. And then she used the notation stating that 'b' was black and 'r' for red-brown. So if you look at these data, you obviously see that they are somehow incompatible.
So, it really took quite some time for Luise to find out and understand the different approaches and to also arrive at a situation where she could merge the two observations into the same format in order to compare it to her theoretical model. So, for Luise it has become clear that it makes sense to put some more effort into the data management, into the planning and deciding what and how data should be gathered in the future. So, this is kind of a data documentation in the first place and we will now look at some recommendation that you could follow as well and when you try to set up scenarios or data management collection scenarios. One idea is to introduce an identifier for each observation that you make. Of course, this is typically something that is auto-generated by the measurement point that you use. But you must think about whether you want to have some things like that and what it can be used for and how it can be used.
The next aspect is that you should, for example, if you state the date when some observation has taken place, that you should agree on the format that you use here. For example, you could agree on the format ISO 8601, specific stating how exactly the date together with the time is represented here. If you do that, it makes it much easier to aggregate the data because you can merge, easily merge the data that has been collected from different places. And you, for example, can aggregate it on a monthly level, or you can even refer to a particular other information like wheather information, for example. The next point is that Luise was interested in distinguishing the number of red-brown versus black squirrels. So, unfortunately, Rachel and Frank have chosen different ways to represent that. So, this is really something where you should impose some vocabulary, some controlled vocabulary. Of course, if you have a standard or something that is agreed upon in your community. If you don't have that, then simply ask your people to follow one approach, for example, saying, well, we use 'b' for black and 'r' for red-brown and not 'b' for brown which then leads to misunderstandings.
Another aspect is something that turns up also in the persistent identifier video. Because if you have your observation points, it might make sense to treat them as data on their own. So, also giving IDs to them and then recording some particular information about them. For example, your exact latitude longitude information, the geographical location of this observation point, maybe also the hardware, the camera that is working within this observation point in order to detect the color of a squirrel. So, this is some kind of the data that the people should agree on, but that's not enough. So, they must also talk about - or ensure - that they have the same understanding of the data. So, this is where metadata comes into play. Of course, it's, in the first place, it's something at least like the column heading of these different columns. So, having ID, having something like date, location, or color. But typically this is even far too short. So, you must have some place where you have some additional information, where you exactly explain how you generate the IDs. For example, whether these are integer numbers or something else, how you record your date, ISO format or not, and also the locations you must agree on. If you register your observation points with some central registry, then you must agree on this procedure and ensure that all the locations are really registered. And the similar thing for the coloring. And that you should use, indeed, a vocabulary that is defined at least in the community or if you don't have that, then decide it for your project how you want to - the different things to be named here. And when Luise looks back at this, she also sees that, well, these observation points that she has used via Rachel and Frank within this project, they also gather the data of the size of the squirrel.
So, while she was not interested in this data, it would of course make sense to record this data as well. Because maybe some other would be interested in exactly this data. And that's exactly what we create here by some follow-up project by Remy Reuse, who wants to look at the evolution of squirrels in entire Europe and is asked to build on the work of Luise and, indeed, he's interested, for example, in the size of the squirrels and that's something that, if Luise has not taken care that this information is preserved as well, can be impossible to build on that.
This has given you some idea of metadata in a very concrete processing but also in a reusing scenario. So, let's look again at the entire research data lifecycle. During planning, of course, you focus on the key elements that your research is about and what data and metadata you want to select here. So, the data gathering is relevant but also maybe your theoretical model, how it is planned, how it is described. Things like that are something that you already have available at the planning level. Also, responsibilities is the kind of a metadata information that Frank Forscher is responsible for Germany and Rachel Research for Great Britain. It's some kind of a metadata that should be available and documented. The next is the point on production, on data collection and -gathering. That's what we looked at in detail within this video. So, typically you have here something like established vocabularies and any additional context information like, for example, the table of observation point locations with any additional detail of the observation measurements. So, this is really something where you can specify your approach and define what information needs to be recorded.
And this is typically done in order to support the analysis phase where you, for example, want to put the things together as we have seen here with Rachel and Frank. And any approach regarding the selection and validation is also some kind of a metadata. So, if you want to only look at the data in either National Park, you must somehow specify how you want to make that selection. You can say, okay, I take the following five observation points or I specify the geographical region and derive which of the observation points reside within this geographical region by comparing latitude and longitude information. You should note down which approach you used in order to look later at your data and maybe understand why some piece of information is wrong because maybe some data is wrong or something like that. So this is also something that supports provenance. Any aggregation approach is also something that is relevant. For example, Luise was only interested in a number of squirrels per month, right? So, we have now seen that within the data model that we looked at today we have an ISO 8601 data format. So, it's rather clear how you arrive at monthly numbers then. And if you record all this kind of information, and how you treat the approaches, and how you're doing that, you also add to your data quality because you make it possible to understand how the data was derived from each other. If you arrive at the level of storage, there are some obvious metadata information, for example, the file format. If you don't know the file format of some file you have really problems in open it and understanding it. But also, for example, the location at the date of the preservation is relevant. Because it helps you to find out whether it makes sense to look at some particular data or if it doesn't make sense because the point that you are interested in has occurred later, for example. And, of course, any additional tooling, for example, that you need in order to correctly interpret the data is something that should be specified. Because otherwise you can't use the data that you have produced there.
Regarding access and reuse, for example, if you publish your data the DOI is certainly something that is important metadata information. Also the repository where your data is published. Or the provenance information, for example, who put the data in the repository, where did it come from, how was it derived. All these things are again relevant here. Another point that is very important - and we will also have a particular video on this - that if you have publications and you have data, it makes sense to note down how they relate. So, what data went into which publications and what publications build on what data? If you have that information easily available then you can easily answer, for example, requests on earlier data that typically arrive because somebody has read your publication. And, of course, the license is also an important metadata information that you produce when you want to publish, for example. Yet, as you can see, there are a lot of metadata occurring throughout the research data lifecycle. And the question is now, of course, how to cope with that, what to do. One recommended good practice is that you make use of a data management plan.
That is something that we detail out in different videos on a generic idea of a Data Management Plan (DMP), as well as the particular contents. The core idea that you take here is, that it's kind of a documentation of your data management. But not only your data management, also your metadata management. And this concerns any phase of the entire research data lifecycle. And typically, a data management plan evolves over time. So, start simple and in the best case in the end you have an entire complete documentation of your data and metadata management within your project and can refer to that document for any question that somebody has, for example, if Remy wants to reuse the data of Luise for his new project. So, in our set up it has become clear that Luise is really thinking that it's a good idea to put more effort into the data management beforehand in order to have less problems within the project but also after the project has been ended. Because if you look at the metadata and store metadata, it helps you maintain your research data, it helps to merge them, for example, it helps to find your data again, for example, the different regions that you have looked at.
You also understand your research data, for example, looking at the controlled vocabulary you simply know, okay, 'r' is red-brown, 'b' is black. So, that's how everything is treated within this project. And there's typically, hopefully, one place where you can look up things like that. So, altogether it helps you use your research data but also if you want to hand it over to others, for example, if you publish it or if others ask for your data, then it's much easier for them to reuse your data. So, all the metadata management, even though it sounds a little bit abstract, is really something that provides you with some additional information, some context information that is needed in order to correctly interpret and use your data.
If you have any more questions on metadata or metadata management, just contact us via the service desk. Thank you!
Quiz
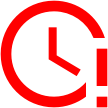
Time is Up!
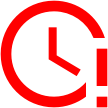
Time’s up
Further Information
Metadata standards directory:
A comprehensive list of metadata standards with short description and further links.
https://rd-alliance.github.io/metadata-directory/
Metadata and describing data:
A short description on the topic of metadata with examples and further links.
https://data.research.cornell.edu/content/writing-metadata
Metadata Guide by Australian Research Data Commons (ARDC):
This Guide is intended to provide a simple generic working-level view of the needs, issues, and processes around metadata collection and creation as it relates to research data.
Citation
FAIR Data Austria (2021). “Metadata”. In: Research Data Management Open Educational Resources Collection. (https://fair-office.at/index.php/metadaten/?lang=en).
License: CC BY 4.0 unless otherwise stated.